|
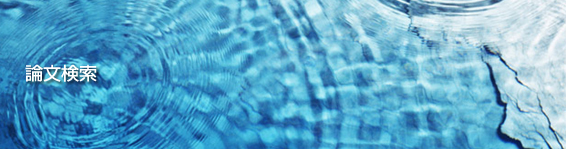
ホーム > 学会誌「EICA」 > 論文検索 > |
|
内容 |
論文 |
巻 |
27巻2/3号2022年
|
Page |
94
|
題名 |
ニューラルネットワークを活用した凝集剤注入率の予測技術の開発
|
Title |
Prediction Model for Flocculant Dosage using AI |
著者 |
渡部亜由美,三宮 豊,横井浩人,中村信幸 |
Authors |
Ayumi Watanabe, Yutaka Sangu, Hiroto Yokoi and Nobuyuki Nakamura |
著者表記 |
(株)日立製作所 |
著者表記(英) |
Hitachi, Ltd. |
著者勤務先名 |
|
Office name |
|
著者所属名 |
|
キーワード |
浄水場,凝集剤,近似式導入ニューラルネットワーク,注入率予測,運転管理支援 |
Key Words |
water treatment, flocculants, neural network, flocculant dosage prediction, operation management support |
概要 |
浄水場の運転管理業務を対象に,凝集剤注入率決定のための予測モデルを開発した。ニューラルネットワーク(NN)は,データを基に運転員の操作実績をモデル化できる一方,学習したデータ範囲外の条件で予測モデル精度が低下する課題がある。そこで,NN と近似式を組み合わせた近似式導入NNを開発し,精度を評価した。学習データ範囲外の条件で,平均絶対誤差は全結合型NNの20.7mg/Lよりも改善し,3.8mg/Lとなった。また,近似式の係数を用いて水温などの入力が凝集剤注入率の予測結果に与える影響を評価し,従来知見と一致することが確認できた。
|
Abstract |
A predictive model for the flocculant dosage was developed for the control of water purification plants. Neural Network (NN), while it is possible to model the operation by workers based on the data, there is a problem prediction model accuracy is lowered under the conditions outside the learning data range. Therefore, an approximation-formula-based NN was built and evaluated the accuracy. Under conditions outside of the learning data range, the approximation-formula-based NN showed higher accuracy (3.8mg/L as mean absolute error) compared to fully-connected NN(20.7mg/L as mean absolute error). In addition, using the approximation-formula-based NN, we visualized the influence of input on the coefficient of approximation formula or prediction. It was confirmed that trends of influences matched with operator knowledges. |
|
|
 |
|
|
◆全文PDFファイルのお申込みの前に
年会費5,000円にて本誌の購読と論文全誌のWeb閲覧がご利用頂けます。
ご入会は、こちらからお願いします。 |
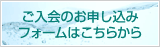 |
|
|
|
この論文は、無料公開されています。 ダウンロードは、右のリンクからどうぞ。
(注)Internet Explorerをお使いの場合、正しいファイル名でダウンロードされない場合があります。 |
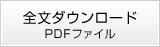 |
|
|
 |
|
|